Dr Paul Brindley
Mapping neighbourhoods
My research provides a framework for quantitative data analysis which allows decision makers to model and interpret phenomena with increased realism. This is achieved through extracting different perspectives found by searching the web. As individuals, we frequently perceive and interpret things (geographic objects, social phenomena and processes) in various ways. In contrast, however, for the purposes of quantitative analysis, objects and events are typically handled as discrete boolean entities with clear boundaries and absolute consensus. Given that the majority of information contains some geospatial reference, the web is a promising source of content to establish a broader range of perspectives, in order to generate fuzzy/probabilistic output.
For example, my thesis provided a new solution to the problem of mapping subjective neighbourhoods. The innovative method identified and extracted neighbourhood names within postal addresses on the web in order to map the vague and frequently overlapping neighbourhood boundaries. Kernel Density Estimation (KDE) surfaces are constructed to form continuous surfaces - as shown in Figure 1.
Back to Paul's Research page
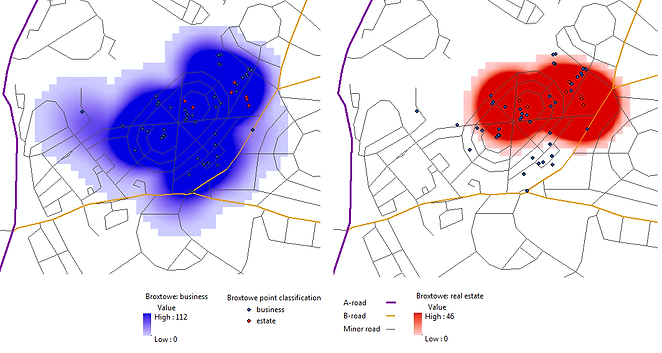
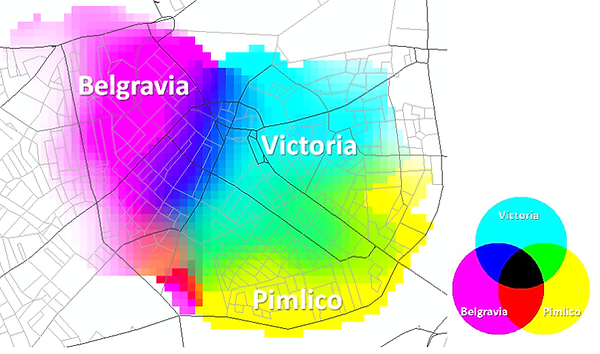
Figure 2: Three neighbourhoods in London
Figure 3: Business & real estate views of Broxtowe, Nottingham
Areas of complete agreement are shown by the 3 subtractive primary colours, whilst overlap in neighbourhood extents are shown by a mixture of the primary colours
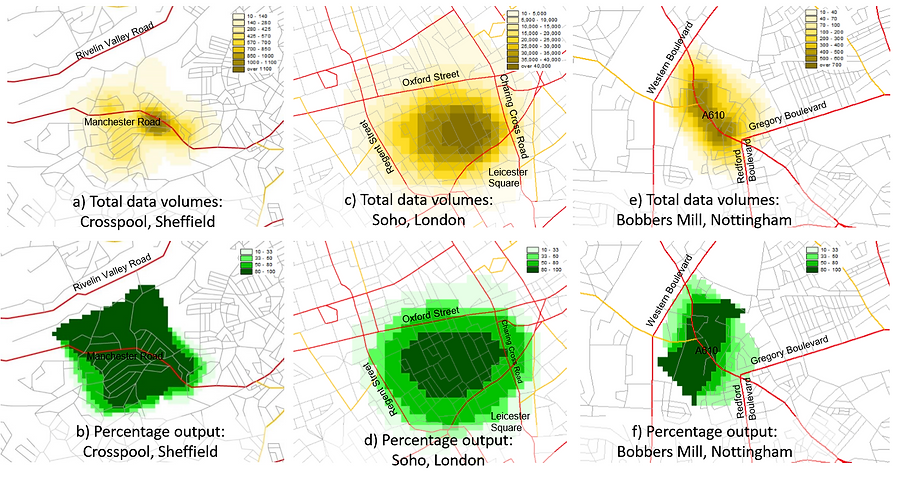
It is then possible to explore the degree to which certain neighbourhoods may overlap. An example of three co-existing neighbourhoods in London is shown in Figure 2. Applying a rule based categorisation of the source URL yielded different data groups, in order to distinguish between a real estate view of neighbourhoods from business source perspectives (as shown in Figure 3).
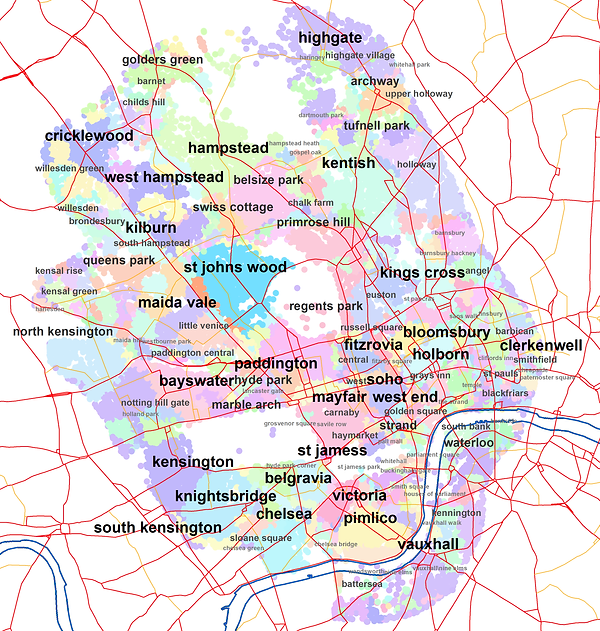
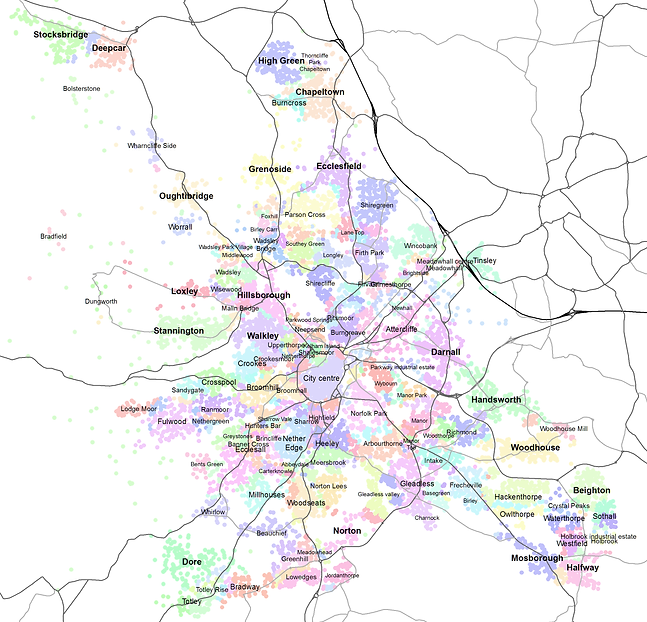
Figure 4: Neighbourhoods for Camden and Westminster, London
Figure 5: Neighbourhoods for Sheffield
In contrast to the individual neighbourhood grids within Figure 1, Figures 4 and 5 illustrate the output when KDE surfaces are allocated back to postcode locations - where each combination of neighbourhood is shown in a different colour (for example in the north-west corner of Sheffield: areas defined as Stocksbridge are coloured green, areas called Deepcar are in red and those areas that are called either Stocksbridge or Deepcar are coloured blue). In addition, these figures scale the size of the labels by the number of data returns, so that more prominent neighbourhoods exert greater visibility.